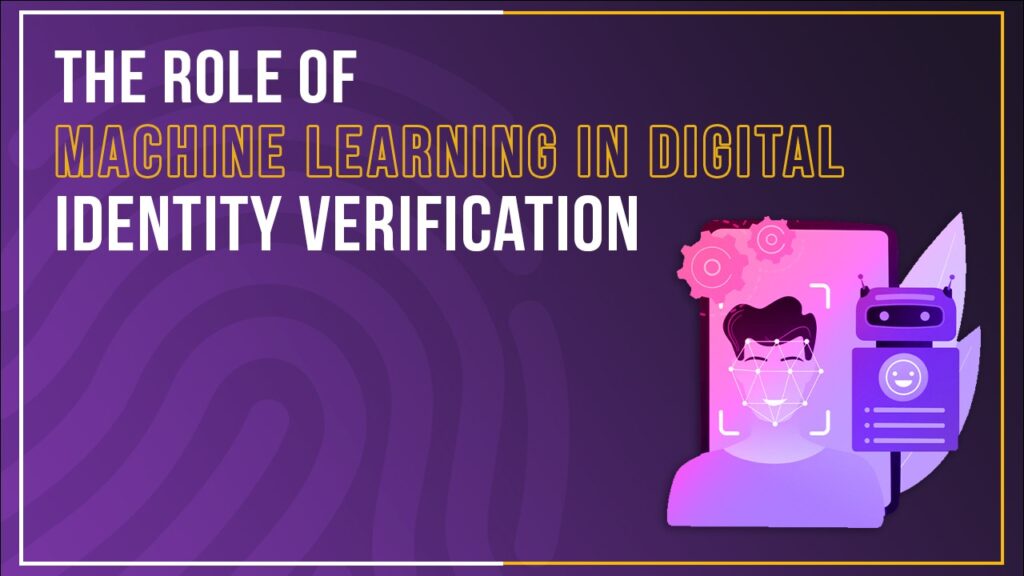
In today's rapidly evolving digital landscape, businesses and organizations are increasingly turning to advanced technologies to enhance security and streamline processes. One of the most critical aspects of online security is identity verification, a process that ensures users are who they claim to be. With the rise in cyber threats and fraudulent activities, traditional verification methods are proving to be insufficient. This is where Machine Learning comes into play, revolutionizing the way businesses approach Identity Verification, Fraud Prevention, and Cybersecurity Measures.
Understanding Machine Learning in Identity Verification
Machine learning (ML) is a subset of artificial intelligence (AI) that enables systems to learn from data, recognize patterns, and make decisions with minimal human intervention. In digital identity verification, ML-powered algorithms analyze vast datasets to detect anomalies, flag suspicious activities, and improve the accuracy of authentication processes.
Traditional identity verification methods, such as manual document checks and password-based authentication, are prone to errors and vulnerabilities. Machine learning significantly enhances these processes by leveraging real-time data analysis and predictive modeling to differentiate between genuine users and fraudulent entities.
How Machine Learning Enhances Identity Verification
Automated Document Verification
Machine learning algorithms can instantly verify identity documents such as passports, driver’s licenses, and national ID cards. These algorithms analyze features like font consistency, holograms, and security marks to ensure document authenticity. Unlike manual checks, ML-driven verification is faster, more efficient, and less prone to human error.
Behavioral Biometrics and Risk Assessment
Machine learning enhances fraud prevention by analyzing users' behavioral patterns, such as typing speed, mouse movements, and touchscreen interactions. If a user deviates from their usual behavior, the system can flag the activity as suspicious and trigger additional verification steps. This adaptive authentication method reduces reliance on static credentials like passwords, which are susceptible to hacking.
Liveness Detection and Biometric Authentication
One of the most significant advancements in identity verification is the use of biometric data, such as facial recognition, fingerprints, and voice recognition. ML-powered systems can perform liveness detection, distinguishing between a real person and a spoofing attempt using photos or deepfake videos. This feature is crucial in preventing identity fraud and enhancing cybersecurity measures.
Real-Time Fraud Detection and Prevention
Fraudsters constantly evolve their techniques to bypass traditional security measures. Machine learning models, trained on vast datasets of fraudulent behaviors, can detect and prevent fraud in real time. These systems continuously learn from new fraud patterns, allowing businesses to stay ahead of cybercriminals.
For example, ML-based fraud detection systems analyze transaction history, IP addresses, device fingerprints, and geolocation data to identify suspicious activities. If an anomaly is detected, the system can take proactive measures such as blocking the transaction or requesting additional verification.
Reducing False Positives and Enhancing User Experience
One of the challenges of traditional identity verification systems is the high rate of false positives, where legitimate users are mistakenly flagged as suspicious. Machine learning minimizes these occurrences by refining risk assessment models over time. This leads to a more seamless user experience, reducing unnecessary friction while maintaining robust security.
The Role of Machine Learning in Fraud Prevention
Machine learning plays a vital role in fraud prevention by identifying and mitigating risks associated with digital transactions and online interactions. Here’s how:
- Adaptive Learning: ML models continuously evolve by learning from new fraud patterns, making them highly effective in detecting emerging threats.
- Anomaly Detection: ML algorithms analyze user behavior, identifying irregular activities that deviate from normal patterns.
- Predictive Analytics: By assessing past fraud cases, ML can predict potential threats and take preventive actions before they occur.
- Multi-Factor Authentication (MFA): ML enhances MFA by determining the level of authentication required based on real-time risk assessments.
Cybersecurity Measures Powered by Machine Learning
In addition to identity verification and fraud prevention, machine learning strengthens cybersecurity measures by providing advanced threat detection and response capabilities. Here are some key areas where ML is making an impact:
- Phishing Detection: ML algorithms analyze email patterns, website URLs, and content to detect phishing attempts.
- Malware Detection: Machine learning models identify malware based on behavioral analysis rather than relying solely on signature-based detection.
- Account Takeover Prevention: ML monitors login behaviors and access patterns to detect and prevent unauthorized account takeovers.
- Threat Intelligence: Machine learning aggregates data from various sources to provide real-time insights into emerging cyber threats.
The Future of Machine Learning in Identity Verification
As technology advances, machine learning will continue to redefine identity verification and fraud prevention strategies. Future developments may include:
- Deep Learning for Enhanced Facial Recognition: Improved accuracy in distinguishing real users from deepfake attacks.
- Blockchain Integration: Combining ML with blockchain technology to create decentralized identity verification systems.
- AI-Powered Chatbots for Secure Customer Interactions: Automating identity verification through conversational AI while ensuring compliance with security protocols.
- Privacy-Preserving Machine Learning: Enhancing data security by enabling identity verification without exposing sensitive user information.
What to Expect?
Machine learning is revolutionizing identity verification, fraud prevention, and cybersecurity measures by providing intelligent, data-driven solutions that enhance security while improving user experience. Businesses that adopt ML-powered identity verification systems can significantly reduce fraud risks, streamline authentication processes, and build trust with their users.
As cyber threats continue to evolve, embracing machine learning is not just an option but a necessity for organizations looking to safeguard their digital ecosystems. By leveraging the power of machine learning, businesses can stay ahead of fraudsters, protect user identities, and create a more secure digital environment for all.